November saw a lot of changes in TOP-10 of our LLM Benchmark. It also saw a few changes in how we build LLM-driven products. Let’s get started.
Update: Claude Sonnet 3.5 v2 - Small capability improvement and great PDF capability
GPT-4o from November 20 - TOP 3!
Qwen 2.5 Coder 32B Instruct - mediocre but pushes SotA!
Qwen QwQ 32B Preview - too smart for its own good
Gemini Experimental 1121 - decent, but hard to get
Plans for LLM Benchmarks v2 - focus on cases and capabilities
Text-to-SQL Benchmark
LLM Benchmarks | November 2024
The benchmarks evaluate the models in terms of their suitability for digital product development. The higher the score, the better.
☁️ - Cloud models with proprietary license
✅ - Open source models that can be run locally without restrictions
🦙 - Local models with Llama license
Can the model generate code and help with programming?
The estimated cost of running the workload. For cloud-based models, we calculate the cost according to the pricing. For on-premises models, we estimate the cost based on GPU requirements for each model, GPU rental cost, model speed, and operational overhead.
How well does the model support work with product catalogs and marketplaces?
How well can the model work with large documents and knowledge bases?
Can the model easily interact with external APIs, services and plugins?
How well can the model support marketing activities, e.g. brainstorming, idea generation and text generation?
How well can the model reason and draw conclusions in a given context?
The "Speed" column indicates the estimated speed of the model in requests per second (without batching). The higher the speed, the better.
Model | Code | Crm | Docs | Integrate | Marketing | Reason | Final | cost | Speed |
---|---|---|---|---|---|---|---|---|---|
1. GPT o1-preview v1/2024-09-12 ☁️ | 95 | 92 | 94 | 95 | 88 | 87 | 92 | 52.32 € | 0.08 rps |
2. GPT o1-mini v1/2024-09-12 ☁️ | 93 | 96 | 94 | 83 | 82 | 87 | 89 | 8.15 € | 0.16 rps |
3. GPT-4o v3/2024-11-20 ☁️ | 86 | 97 | 94 | 95 | 88 | 72 | 89 | 0.63 € | 1.14 rps |
4. GPT-4o v1/2024-05-13 ☁️ | 90 | 96 | 100 | 92 | 78 | 74 | 88 | 1.21 € | 1.44 rps |
5. Google Gemini 1.5 Pro v2 ☁️ | 86 | 97 | 94 | 99 | 78 | 74 | 88 | 1.00 € | 1.18 rps |
6. GPT-4 Turbo v5/2024-04-09 ☁️ | 86 | 99 | 98 | 96 | 88 | 43 | 85 | 2.45 € | 0.84 rps |
7. Google Gemini Exp 1121 ☁️ | 70 | 97 | 97 | 95 | 72 | 72 | 84 | 0.89 € | 0.49 rps |
8. GPT-4o v2/2024-08-06 ☁️ | 90 | 84 | 97 | 86 | 82 | 59 | 83 | 0.63 € | 1.49 rps |
9. Google Gemini 1.5 Pro 0801 ☁️ | 84 | 92 | 79 | 100 | 70 | 74 | 83 | 0.90 € | 0.83 rps |
10. Qwen 2.5 72B Instruct ⚠️ | 79 | 92 | 94 | 97 | 71 | 59 | 82 | 0.10 € | 0.66 rps |
11. Llama 3.1 405B Hermes 3🦙 | 68 | 93 | 89 | 98 | 88 | 53 | 81 | 0.54 € | 0.49 rps |
12. Claude 3.5 Sonnet v2 ☁️ | 82 | 97 | 93 | 84 | 71 | 57 | 81 | 0.95 € | 0.09 rps |
13. GPT-4 v1/0314 ☁️ | 90 | 88 | 98 | 73 | 88 | 45 | 80 | 7.04 € | 1.31 rps |
14. X-AI Grok 2 ⚠️ | 63 | 93 | 87 | 90 | 88 | 58 | 80 | 1.03 € | 0.31 rps |
15. GPT-4 v2/0613 ☁️ | 90 | 83 | 95 | 73 | 88 | 45 | 79 | 7.04 € | 2.16 rps |
16. GPT-4o Mini ☁️ | 63 | 87 | 80 | 73 | 100 | 65 | 78 | 0.04 € | 1.46 rps |
17. Claude 3.5 Sonnet v1 ☁️ | 72 | 83 | 89 | 87 | 80 | 58 | 78 | 0.94 € | 0.09 rps |
18. Claude 3 Opus ☁️ | 69 | 88 | 100 | 74 | 76 | 58 | 77 | 4.69 € | 0.41 rps |
19. Meta Llama3.1 405B Instruct🦙 | 81 | 93 | 92 | 75 | 75 | 48 | 77 | 2.39 € | 1.16 rps |
20. GPT-4 Turbo v4/0125-preview ☁️ | 66 | 97 | 100 | 83 | 75 | 43 | 77 | 2.45 € | 0.84 rps |
21. Google LearnLM 1.5 Pro Experimental ⚠️ | 48 | 97 | 85 | 96 | 64 | 72 | 77 | 0.31 € | 0.83 rps |
22. GPT-4 Turbo v3/1106-preview ☁️ | 66 | 75 | 98 | 73 | 88 | 60 | 76 | 2.46 € | 0.68 rps |
23. Qwen 2.5 32B Coder Instruct ⚠️ | 43 | 94 | 98 | 98 | 76 | 46 | 76 | 0.05 € | 0.82 rps |
24. DeepSeek v2.5 236B ⚠️ | 57 | 80 | 91 | 80 | 88 | 57 | 75 | 0.03 € | 0.42 rps |
25. Meta Llama 3.1 70B Instruct f16🦙 | 74 | 89 | 90 | 75 | 75 | 48 | 75 | 1.79 € | 0.90 rps |
26. Google Gemini 1.5 Flash v2 ☁️ | 64 | 96 | 89 | 76 | 81 | 44 | 75 | 0.06 € | 2.01 rps |
27. Google Gemini 1.5 Pro 0409 ☁️ | 68 | 97 | 96 | 80 | 75 | 26 | 74 | 0.95 € | 0.59 rps |
28. Meta Llama 3 70B Instruct🦙 | 81 | 83 | 84 | 67 | 81 | 45 | 73 | 0.06 € | 0.85 rps |
29. GPT-3.5 v2/0613 ☁️ | 68 | 81 | 73 | 87 | 81 | 50 | 73 | 0.34 € | 1.46 rps |
30. Mistral Large 123B v2/2407 ☁️ | 68 | 79 | 68 | 75 | 75 | 70 | 72 | 0.57 € | 1.02 rps |
31. Google Gemini Flash 1.5 8B ☁️ | 70 | 93 | 78 | 67 | 76 | 48 | 72 | 0.01 € | 1.19 rps |
32. Google Gemini 1.5 Pro 0514 ☁️ | 73 | 96 | 79 | 100 | 25 | 60 | 72 | 1.07 € | 0.92 rps |
33. Google Gemini 1.5 Flash 0514 ☁️ | 32 | 97 | 100 | 76 | 72 | 52 | 72 | 0.06 € | 1.77 rps |
34. Google Gemini 1.0 Pro ☁️ | 66 | 86 | 83 | 79 | 88 | 28 | 71 | 0.37 € | 1.36 rps |
35. Meta Llama 3.2 90B Vision🦙 | 74 | 84 | 87 | 77 | 71 | 32 | 71 | 0.23 € | 1.10 rps |
36. GPT-3.5 v3/1106 ☁️ | 68 | 70 | 71 | 81 | 78 | 58 | 71 | 0.24 € | 2.33 rps |
37. Claude 3.5 Haiku ☁️ | 52 | 80 | 72 | 75 | 75 | 68 | 70 | 0.32 € | 1.24 rps |
38. GPT-3.5 v4/0125 ☁️ | 63 | 87 | 71 | 77 | 78 | 43 | 70 | 0.12 € | 1.43 rps |
39. Cohere Command R+ ☁️ | 63 | 80 | 76 | 72 | 70 | 58 | 70 | 0.83 € | 1.90 rps |
40. Mistral Large 123B v3/2411 ☁️ | 68 | 75 | 64 | 76 | 82 | 51 | 70 | 0.56 € | 0.66 rps |
41. Qwen1.5 32B Chat f16 ⚠️ | 70 | 90 | 82 | 76 | 78 | 20 | 69 | 0.97 € | 1.66 rps |
42. Gemma 2 27B IT ⚠️ | 61 | 72 | 87 | 74 | 89 | 32 | 69 | 0.07 € | 0.90 rps |
43. Mistral 7B OpenChat-3.5 v3 0106 f16 ✅ | 68 | 87 | 67 | 74 | 88 | 25 | 68 | 0.32 € | 3.39 rps |
44. Meta Llama 3 8B Instruct f16🦙 | 79 | 62 | 68 | 70 | 80 | 41 | 67 | 0.32 € | 3.33 rps |
45. Gemma 7B OpenChat-3.5 v3 0106 f16 ✅ | 63 | 67 | 84 | 58 | 81 | 46 | 67 | 0.21 € | 5.09 rps |
46. GPT-3.5-instruct 0914 ☁️ | 47 | 92 | 69 | 69 | 88 | 33 | 66 | 0.35 € | 2.15 rps |
47. GPT-3.5 v1/0301 ☁️ | 55 | 82 | 69 | 81 | 82 | 26 | 66 | 0.35 € | 4.12 rps |
48. Llama 3 8B OpenChat-3.6 20240522 f16 ✅ | 76 | 51 | 76 | 65 | 88 | 38 | 66 | 0.28 € | 3.79 rps |
49. Mistral 7B OpenChat-3.5 v1 f16 ✅ | 58 | 72 | 72 | 71 | 88 | 33 | 66 | 0.49 € | 2.20 rps |
50. Mistral 7B OpenChat-3.5 v2 1210 f16 ✅ | 63 | 73 | 72 | 66 | 88 | 30 | 65 | 0.32 € | 3.40 rps |
51. Qwen 2.5 7B Instruct ⚠️ | 48 | 77 | 80 | 68 | 69 | 47 | 65 | 0.07 € | 1.25 rps |
52. Starling 7B-alpha f16 ⚠️ | 58 | 66 | 67 | 73 | 88 | 34 | 64 | 0.58 € | 1.85 rps |
53. Mistral Nemo 12B v1/2407 ☁️ | 54 | 58 | 51 | 99 | 75 | 49 | 64 | 0.03 € | 1.22 rps |
54. Meta Llama 3.2 11B Vision🦙 | 70 | 71 | 65 | 70 | 71 | 36 | 64 | 0.04 € | 1.49 rps |
55. Llama 3 8B Hermes 2 Theta🦙 | 61 | 73 | 74 | 74 | 85 | 16 | 64 | 0.05 € | 0.55 rps |
56. Claude 3 Haiku ☁️ | 64 | 69 | 64 | 75 | 75 | 35 | 64 | 0.08 € | 0.52 rps |
57. Yi 1.5 34B Chat f16 ⚠️ | 47 | 78 | 70 | 74 | 86 | 26 | 64 | 1.18 € | 1.37 rps |
58. Liquid: LFM 40B MoE ⚠️ | 72 | 69 | 65 | 63 | 82 | 24 | 63 | 0.00 € | 1.45 rps |
59. Meta Llama 3.1 8B Instruct f16🦙 | 57 | 74 | 62 | 74 | 74 | 32 | 62 | 0.45 € | 2.41 rps |
60. Qwen2 7B Instruct f32 ⚠️ | 50 | 81 | 81 | 61 | 66 | 31 | 62 | 0.46 € | 2.36 rps |
61. Claude 3 Sonnet ☁️ | 72 | 41 | 74 | 74 | 78 | 28 | 61 | 0.95 € | 0.85 rps |
62. Mistral Small v3/2409 ☁️ | 43 | 75 | 71 | 74 | 75 | 26 | 61 | 0.06 € | 0.81 rps |
63. Mistral Pixtral 12B ✅ | 53 | 69 | 73 | 63 | 64 | 40 | 60 | 0.03 € | 0.83 rps |
64. Mixtral 8x22B API (Instruct) ☁️ | 53 | 62 | 62 | 97 | 75 | 7 | 59 | 0.17 € | 3.12 rps |
65. Anthropic Claude Instant v1.2 ☁️ | 58 | 75 | 65 | 77 | 65 | 16 | 59 | 2.10 € | 1.49 rps |
66. Codestral Mamba 7B v1 ✅ | 53 | 66 | 51 | 97 | 71 | 17 | 59 | 0.30 € | 2.82 rps |
67. Inflection 3 Productivity ⚠️ | 46 | 59 | 39 | 70 | 79 | 61 | 59 | 0.92 € | 0.17 rps |
68. Anthropic Claude v2.0 ☁️ | 63 | 52 | 55 | 67 | 84 | 34 | 59 | 2.19 € | 0.40 rps |
69. Cohere Command R ☁️ | 45 | 66 | 57 | 74 | 84 | 27 | 59 | 0.13 € | 2.50 rps |
70. Qwen1.5 7B Chat f16 ⚠️ | 56 | 81 | 60 | 56 | 60 | 36 | 58 | 0.29 € | 3.76 rps |
71. Mistral Large v1/2402 ☁️ | 37 | 49 | 70 | 83 | 84 | 25 | 58 | 0.58 € | 2.11 rps |
72. Microsoft WizardLM 2 8x22B ⚠️ | 48 | 76 | 79 | 59 | 62 | 22 | 58 | 0.13 € | 0.70 rps |
73. Qwen1.5 14B Chat f16 ⚠️ | 50 | 58 | 51 | 72 | 84 | 22 | 56 | 0.36 € | 3.03 rps |
74. MistralAI Ministral 8B ✅ | 56 | 55 | 41 | 82 | 68 | 30 | 55 | 0.02 € | 1.02 rps |
75. Anthropic Claude v2.1 ☁️ | 29 | 58 | 59 | 78 | 75 | 32 | 55 | 2.25 € | 0.35 rps |
76. Mistral 7B OpenOrca f16 ☁️ | 54 | 57 | 76 | 36 | 78 | 27 | 55 | 0.41 € | 2.65 rps |
77. MistralAI Ministral 3B ✅ | 50 | 48 | 39 | 89 | 60 | 41 | 54 | 0.01 € | 1.02 rps |
78. Llama2 13B Vicuna-1.5 f16🦙 | 50 | 37 | 55 | 62 | 82 | 37 | 54 | 0.99 € | 1.09 rps |
79. Mistral 7B Instruct v0.1 f16 ☁️ | 34 | 71 | 69 | 63 | 62 | 23 | 54 | 0.75 € | 1.43 rps |
80. Meta Llama 3.2 3B🦙 | 52 | 71 | 66 | 71 | 44 | 14 | 53 | 0.01 € | 1.25 rps |
81. Google Recurrent Gemma 9B IT f16 ⚠️ | 58 | 27 | 71 | 64 | 56 | 23 | 50 | 0.89 € | 1.21 rps |
82. Codestral 22B v1 ✅ | 38 | 47 | 44 | 84 | 66 | 13 | 49 | 0.06 € | 4.03 rps |
83. Qwen: QwQ 32B Preview ⚠️ | 43 | 32 | 74 | 52 | 48 | 40 | 48 | 0.05 € | 0.63 rps |
84. Llama2 13B Hermes f16🦙 | 50 | 24 | 37 | 75 | 60 | 42 | 48 | 1.00 € | 1.07 rps |
85. IBM Granite 34B Code Instruct f16 ☁️ | 63 | 49 | 34 | 67 | 57 | 7 | 46 | 1.07 € | 1.51 rps |
86. Meta Llama 3.2 1B🦙 | 32 | 40 | 33 | 53 | 68 | 51 | 46 | 0.02 € | 1.69 rps |
87. Mistral Small v2/2402 ☁️ | 33 | 42 | 45 | 88 | 56 | 8 | 46 | 0.06 € | 3.21 rps |
88. Mistral Small v1/2312 (Mixtral) ☁️ | 10 | 67 | 63 | 65 | 56 | 8 | 45 | 0.06 € | 2.21 rps |
89. DBRX 132B Instruct ⚠️ | 43 | 39 | 43 | 74 | 59 | 10 | 45 | 0.26 € | 1.31 rps |
90. NVIDIA Llama 3.1 Nemotron 70B Instruct🦙 | 68 | 54 | 25 | 72 | 28 | 21 | 45 | 0.09 € | 0.53 rps |
91. Mistral Medium v1/2312 ☁️ | 41 | 43 | 44 | 59 | 62 | 12 | 44 | 0.81 € | 0.35 rps |
92. Microsoft WizardLM 2 7B ⚠️ | 53 | 34 | 42 | 66 | 53 | 13 | 43 | 0.02 € | 0.89 rps |
93. Llama2 13B Puffin f16🦙 | 37 | 15 | 44 | 67 | 56 | 39 | 43 | 4.70 € | 0.23 rps |
94. Mistral Tiny v1/2312 (7B Instruct v0.2) ☁️ | 22 | 47 | 59 | 53 | 62 | 8 | 42 | 0.05 € | 2.39 rps |
95. Gemma 2 9B IT ⚠️ | 45 | 25 | 47 | 36 | 68 | 13 | 39 | 0.02 € | 0.88 rps |
96. Meta Llama2 13B chat f16🦙 | 22 | 38 | 17 | 65 | 75 | 6 | 37 | 0.75 € | 1.44 rps |
97. Mistral 7B Zephyr-β f16 ✅ | 37 | 34 | 46 | 62 | 29 | 4 | 35 | 0.46 € | 2.34 rps |
98. Meta Llama2 7B chat f16🦙 | 22 | 33 | 20 | 62 | 50 | 18 | 34 | 0.56 € | 1.93 rps |
99. Mistral 7B Notus-v1 f16 ⚠️ | 10 | 54 | 25 | 60 | 48 | 4 | 33 | 0.75 € | 1.43 rps |
100. Orca 2 13B f16 ⚠️ | 18 | 22 | 32 | 29 | 67 | 20 | 31 | 0.95 € | 1.14 rps |
101. Mistral 7B Instruct v0.2 f16 ☁️ | 11 | 30 | 54 | 25 | 58 | 8 | 31 | 0.96 € | 1.12 rps |
102. Mistral 7B v0.1 f16 ☁️ | 0 | 9 | 48 | 63 | 52 | 12 | 31 | 0.87 € | 1.23 rps |
103. Google Gemma 2B IT f16 ⚠️ | 33 | 28 | 16 | 47 | 15 | 20 | 27 | 0.30 € | 3.54 rps |
104. Microsoft Phi 3 Medium 4K Instruct 14B f16 ⚠️ | 5 | 34 | 30 | 32 | 47 | 8 | 26 | 0.82 € | 1.32 rps |
105. Orca 2 7B f16 ⚠️ | 22 | 0 | 26 | 26 | 52 | 4 | 22 | 0.78 € | 1.38 rps |
106. Google Gemma 7B IT f16 ⚠️ | 0 | 0 | 0 | 6 | 62 | 0 | 11 | 0.99 € | 1.08 rps |
107. Meta Llama2 7B f16🦙 | 0 | 5 | 22 | 3 | 28 | 2 | 10 | 0.95 € | 1.13 rps |
108. Yi 1.5 9B Chat f16 ⚠️ | 0 | 4 | 29 | 17 | 0 | 8 | 10 | 1.41 € | 0.76 rps |
Claude 3.5 v2 Update and document extraction in manufacturing
In the october LLM Benchmark we’ve written that Anthropic has achieved a small improvement in the capabilities of its Claude 3.5 v2. That improvement is relatively small, but not enough to put it into the TOP-10.
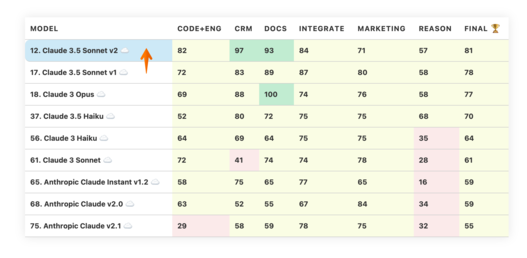
Yet, this Anthropic Claude 3.5 Sonnet v2 is currently our first choice for data extraction projects (e.g. as a part of business automation in manufacturing industries). Why is it so?
For example, imagine that you need to carefully extract product specification data for 20000 electrical components out of 1000 data sheets. These PDFs could include complex tables and even charts. Extracted data could then be used for comparing company products to the products of competitors, offering equivalent components in inline ads or driving supply chain decisions.
Anthropic Claude 3.5 Sonnet v2 has two nice features that work well together:
Native PDF Handling - we can now upload PDF files directly into the API along with the data extraction instructions. Under the hood, the Anthropic API will break the PDF down into pages and upload each page twice: as image and as text. This solution works well enough out of the box to replace previously complicated setups that used dedicated VLMs (Visual Language Models) running on local GPUs.
PDFs can consume a lot of tokens, especially when accompanied with a large system prompt. To speed up the processing, improve accuracy and lower costs we use two-level Prompt caching from Anthropic. This allows us to pay the full cost of PDF tokenisation only once.
Here is how our prompt can look for the data extraction:
System prompt: Your task is to extract product data from the PDF. Here is the schema (large schema) and company context.
Document prompt: Here is the PDF to extract the data from. It has multiple products. (large PDF)
Task: extract product X from the PDF.
This way we can extract multiple products from the single PDF (following the checklist pattern). System prompt (1) and Document prompt (2) will be cached between all extraction requests to the same PDF. System (1) will be cached between all requests for this type of PDF extraction in general.
Whenever a portion of the prompt is cached on the server - it costs less and runs faster. For example, 30-70% faster and 50-90% cheaper, as described in the documentation of Anthropic. In data extraction cases, cost savings tend to be closer to the upper end of that range.
This is how things look in action: 'Cache creation' indicates when part of the prompt is stored in the cache, and 'Cache read' shows when the cached prompt is reused, saving time and money.
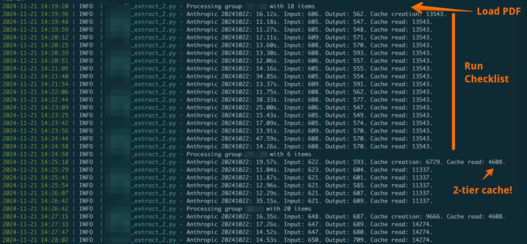
There is a small caveat. Anthropic models don’t have Structured Output capability of OpenAI. So you would think that we can lose two amazing features:
Precise schema following
Ability to hardcode custom chain-of-thought process that will drive LLM through the data extraction process.
However, this is not the case! Structured Output is just an inference capability that drives constrained decoding (token selection) to follow the schema precisely. A capable LLM will be able to extract even a complex structure without it. And while doing so, it will follow chain-of-thought process encoded in the schema definition.
Anthropic Claude 3.5 Sonnet v2 certainly can perform that. And in 5-7% of cases that return slightly invalid schema, we can pass results to GPT-4o for the schema repair.
For reference, here is an example of Structured Output definition from one of the projects (image quality was lowered intentionally).
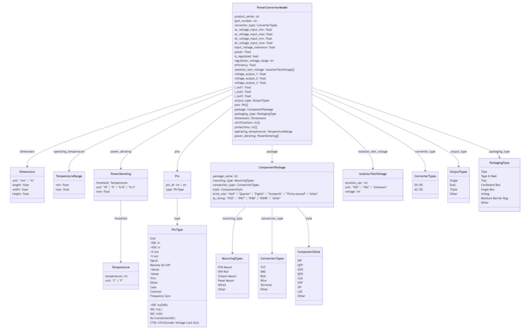
GPT-4o from November 20 - TOP 3
OpenAI didn’t bother to publish a proper announcement for this model (gpt-4o-2024-11-20
in the API). They have just tweeted the update:
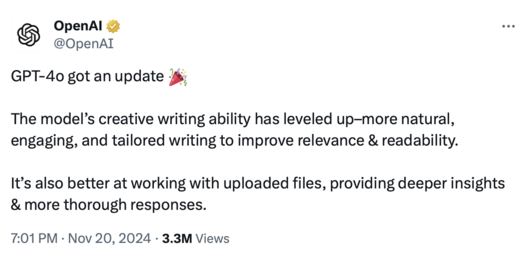
The model deserves a special mention in our benchmarks. Compared to the previous GPT-4o v2/2024-08-06, the model shows noticeable improvement, especially in the Reason category.
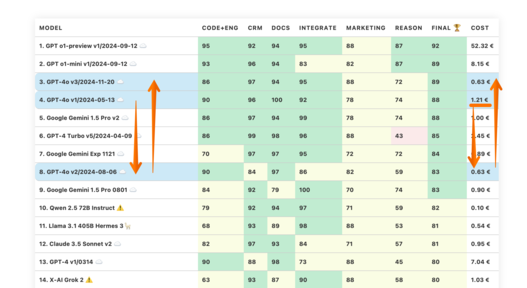
You can also note the usual pattern of OpenAI with the models:
First, they release a new powerful model (GPT-4o v1 in this case)
Then they release the next model in the same family that is much cheaper to run
Finally, they improve the model to be better, while still running at lower costs.
Multiple Qwen models from Alibaba
Qwen 2.5 Coder 32B Instruct is a new model in Qwen family. It will first make you sad and then—glad.
The model itself can be downloaded from HuggingFace and run locally on your hardware.
The sad part is that this coding model performed poorly on our Code+Eng category of tasks. It was able to handle coding tasks, but failed to deal with more complex code review and analysis challenges. Besides, its reasoning is generally quite low - 46.
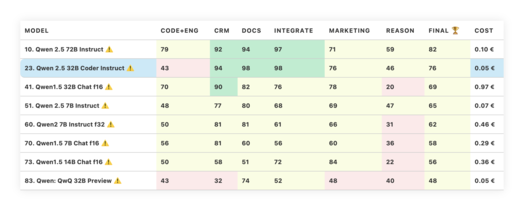
What would you expect from a model that is called “Coder”, right? And actually in coding this model is quite good. This model performed as well as Sonnet 3.5 in coding-only benchmark for complex text-to-SQL tasks (more about that later).
What is so good about this model, then? This coding-oriented model represents a new quality improvement for local models in category “It can run on A100/H100 GPU”!
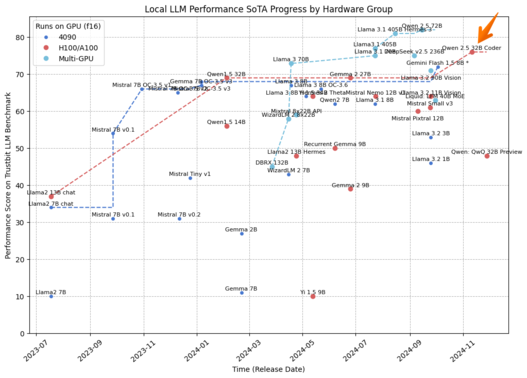
By the way, it is interesting to note that a few other big quality improvements that pushed State of the Art for local models were also driven by Qwen.
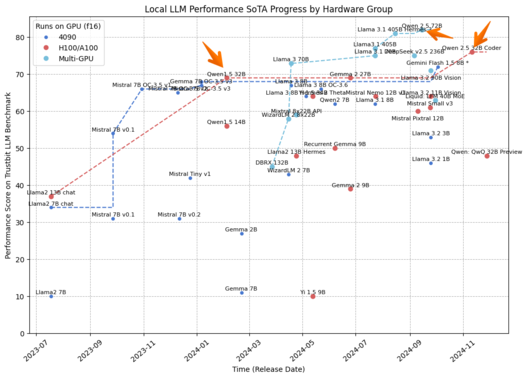
It is also interesting that “o1-killer” from Qwen didn’t score that high on our benchmark. Qwen: QwQ 32B Preview was designed to push state of the art in reasoning capabilities. According to some benchmarks it did succeed. However, it doesn’t look like a fit for product tasks and business automation. Why? It talks too much and doesn’t follow the instructions.
For example, given the prompt below, that is also reinforced by a couple of samples:
You extract product properties from provided text. Respond in format: "number unit" or "N/A" if can't determine. Strip quotes, thousands separators and comments.
The model will tend to start the response this way:
Alright, I've got this text about an electric screwdriver,...
Even the tiny mistral-7b-instruct-f16
would answer precisely something like 1300 rpm
.
This might seem like an unfair comparison for QwQ against a top model o1-preview. o1 has a chance to reason in private before providing its response (it uses reasoning tokens for that).
To make things more fair for the new generations of reasoning models, we will change things a bit in the next major update of our benchmark - models will be allowed to reason before providing an answer. Models that think too much will be natively penalised by their cost and huge latency.
LLM Benchmark v2
We’ve been running current version of the benchmark without major changes for almost a year and a half. Changes were avoided to keep benchmark results comparable between models and test runs.
However, a lot has changed in the landscape since July 2023:
Structured Outputs - allow us to define precise response format and even drive custom chain-of-thought for the complex tasks.
Multi-modal language models can handle images and audio in addition to text input. Image inputs are used heavily in document extraction.
Prompt caching shifts perspective for building RAG systems, running complex checklists or extracting data from a lot of documents.
New reasoning models allow us to push model performance forward by breaking down complex tasks into small steps and then investing (paid) time to think through them.
In addition to that, we’ve gained a lot more insights in building LLM-driven systems and added more cases to our AI portfolio.
It is time for a big refresh. The work on the TIMETOACT GROUP LLM Benchmark v2 has already started. We are expecting to publish the first draft report early next year.
The V2 benchmark will keep the foundations from v1 but will focus more on concrete AI Cases and new model capabilities. More charts are to be expected, too.
Gemini Experimental 1121 - Good, but “unobtanium”
Gemini Experimental 1121 is a new prototype model from Google. It is currently available in test environments like AI Studio or OpenRouter. This model doesn’t push state of the art for Gemini, but proves that the presence of Google in TOP-10 is not a lucky coincidence. It is the third Gemini model to be in TOP-10.
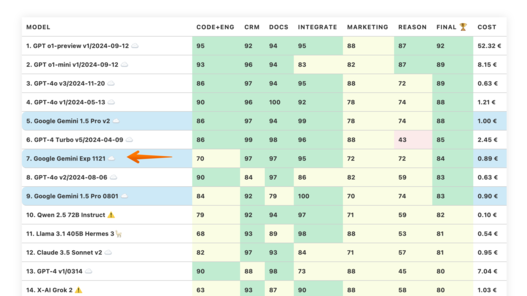
However, this model is currently impossible to use. It is provided for free but is heavily rate limited. It took 3 days and multiple API keys just to run a few hundred evals from our benchmark.
Text-to-SQL Benchmark
Neo4j has published a video from its NODES24 conference about benchmarking different LLMs in text-to-SQL and text-to-Cypher tasks.
An example of a Text-to-SQL task is when an LLM is used to translate human request into a complex query against company SQL database. It is used for self-service reporting. Text-to-Cypher is the same, but runs queries against graph databases like Neo4j.
The research and presentation was done in partnership with two companies from the TIMETOACT GROUP: X-Integrate and TIMETOACT GROUP Austria.
The most important slide of the presentation is the one below. It shows accuracy with which different LLMs have generated queries for a complex database. This DB held information about technical and organisational dependencies in the company for the purposes of risk management.
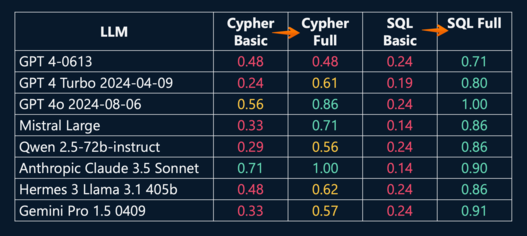
Basic” scores are the scores without any performance optimisations, while “Full” scores employ a range of performance optimisations to boost the accuracy of query generation.
You can learn more about these optimisations (and about the benchmark) by watching the presentation online on YouTube.
Some of these text-to-query tasks will even be included in our upcoming LLM v2 benchmark.
Transform Your Digital Projects with the Best AI Language Models!
Discover the transformative power of the best LLMs and revolutionize your digital products with AI! Stay future-focused, boost efficiency, and gain a clear competitive edge. We help you elevate your business value to the next level.
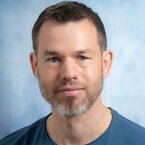
Martin Warnung
martin.warnung@timetoact.at
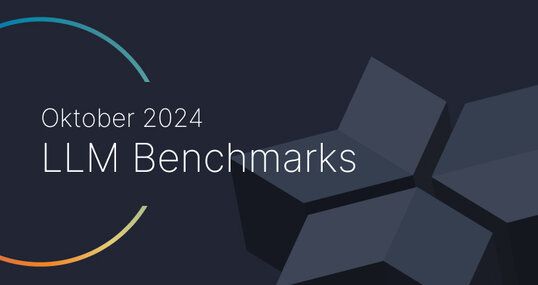
ChatGPT & Co: LLM Benchmarks for October
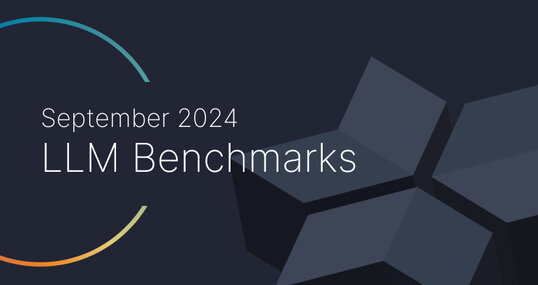
ChatGPT & Co: LLM Benchmarks for September
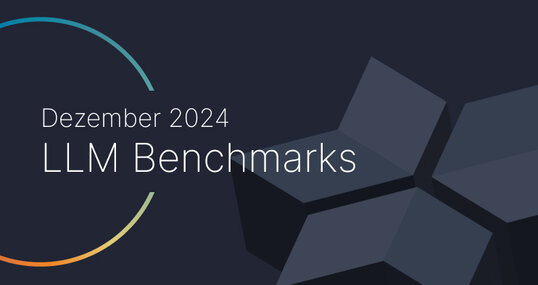
ChatGPT & Co: LLM Benchmarks for December
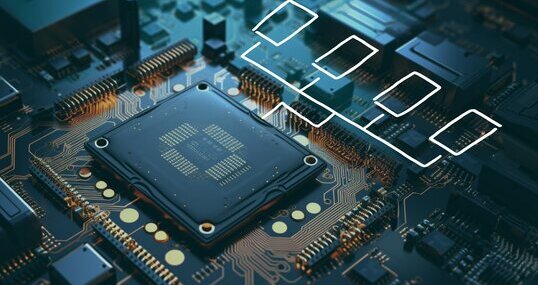
LLM Performance Series: Batching
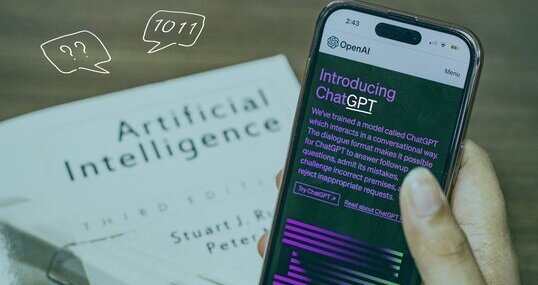
Strategic Impact of Large Language Models
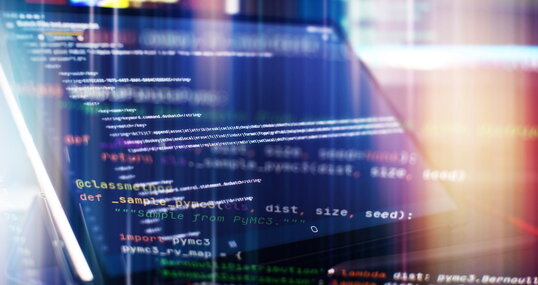
Open-sourcing 4 solutions from the Enterprise RAG Challenge
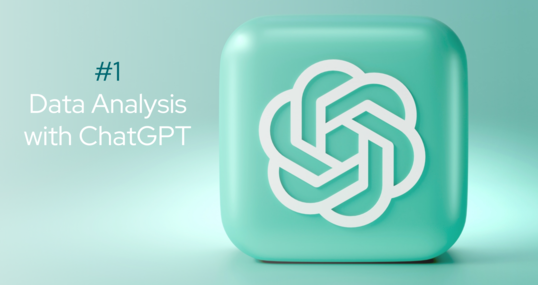
Part 1: Data Analysis with ChatGPT
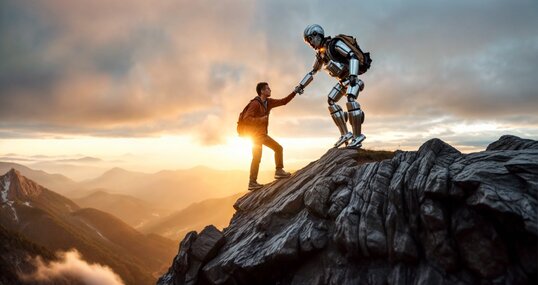
8 tips for developing AI assistants
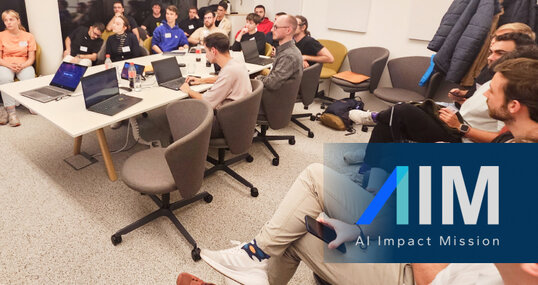
AIM Hackathon 2024: Sustainability Meets LLMs
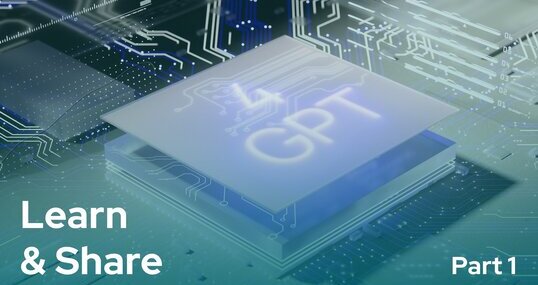
Creating a Social Media Posts Generator Website with ChatGPT
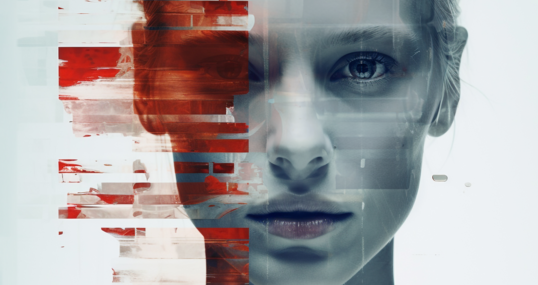
Common Mistakes in the Development of AI Assistants
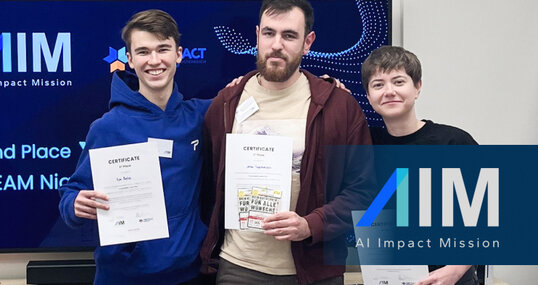
Second Place - AIM Hackathon 2024: Trustpilot for ESG
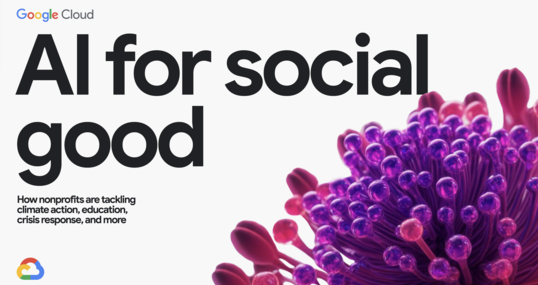
AI for social good
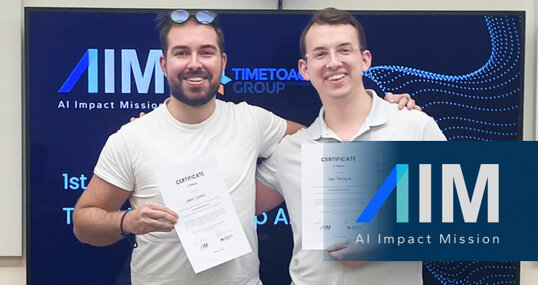
SAM Wins First Prize at AIM Hackathon
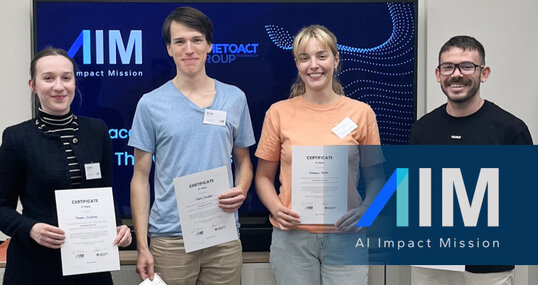
Third Place - AIM Hackathon 2024: The Venturers
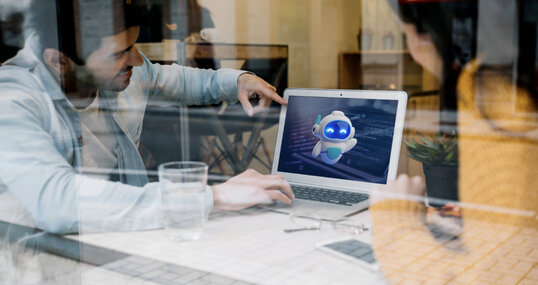
Let's build an Enterprise AI Assistant
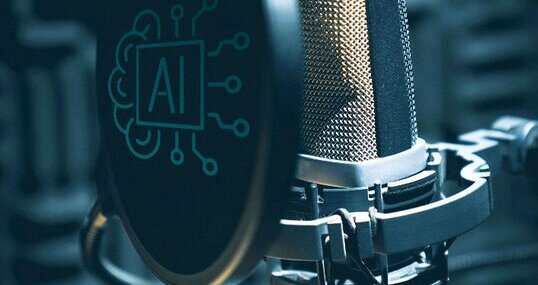
The Intersection of AI and Voice Manipulation
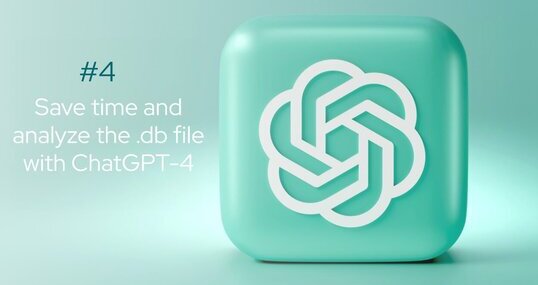
Part 4: Save Time and Analyze the Database File
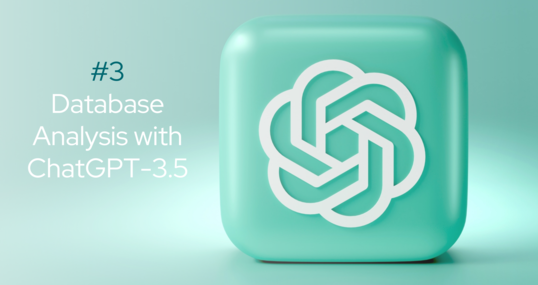
Part 3: How to Analyze a Database File with GPT-3.5
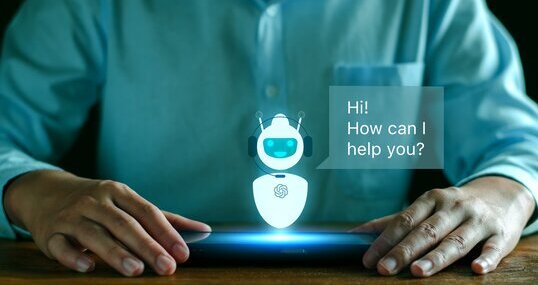
So You are Building an AI Assistant?
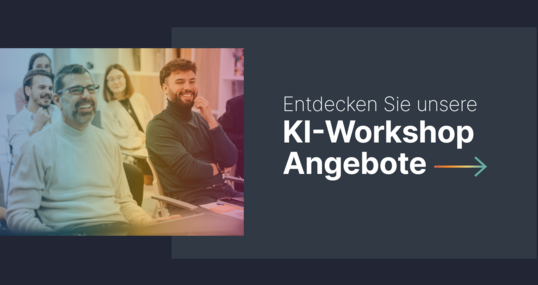
AI Workshops for Companies
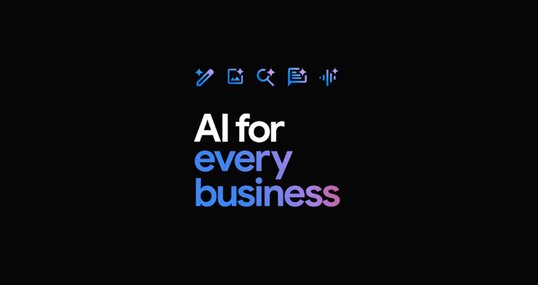
Google Workspace: AI-supported work for every company
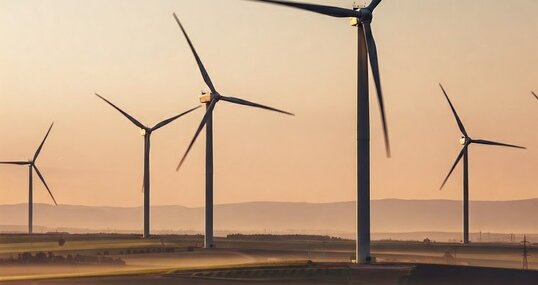
Crisis management & building a sustainable future with AI
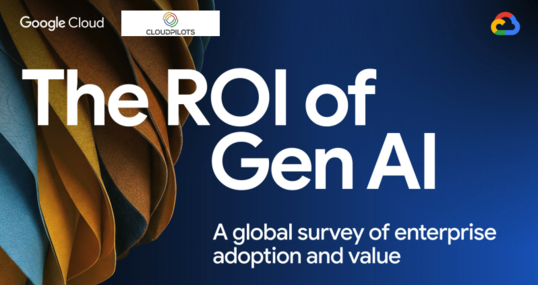
The ROI of Gen AI
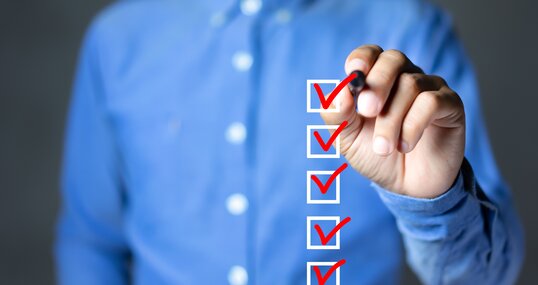
License Management – Everything you need to know
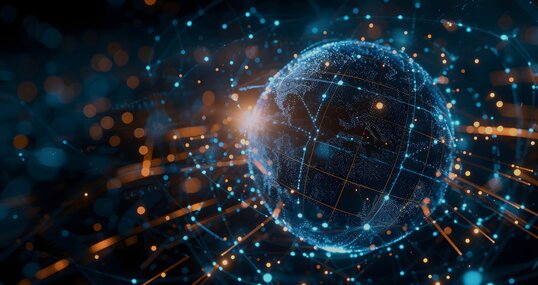
Graph Technology
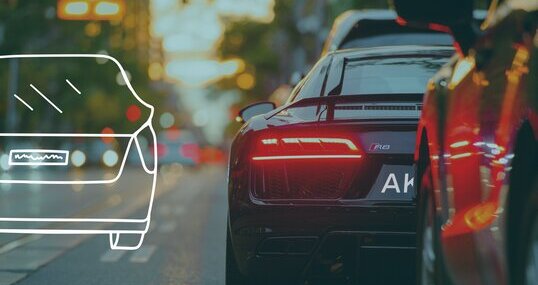
License Plate Detection for Precise Car Distance Estimation
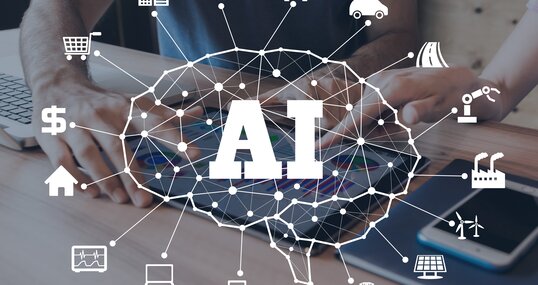
IBM Cloud Pak for Data Accelerator
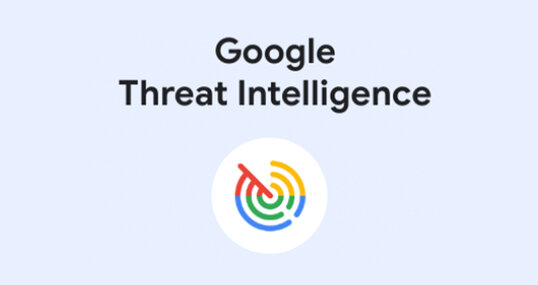
Google Threat Intelligence
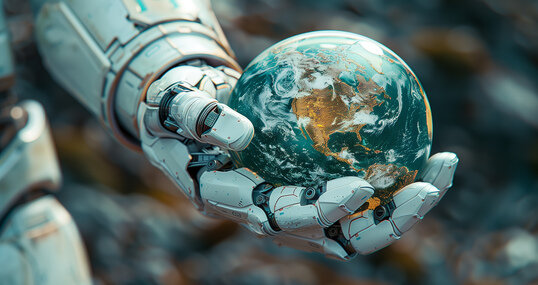
Responsible AI: A Guide to Ethical AI Development

5 Inconvenient Questions when hiring an AI company
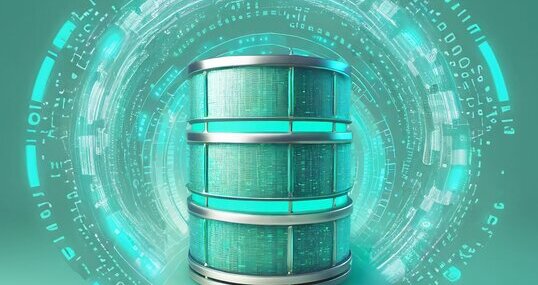
Database Analysis Report
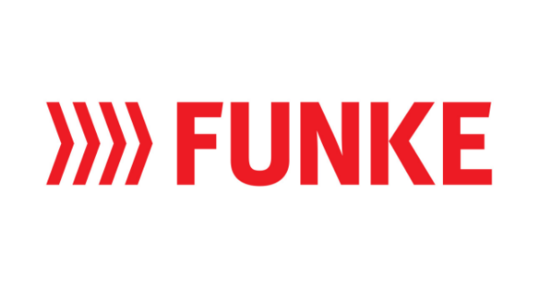
Managed service support for optimal license management
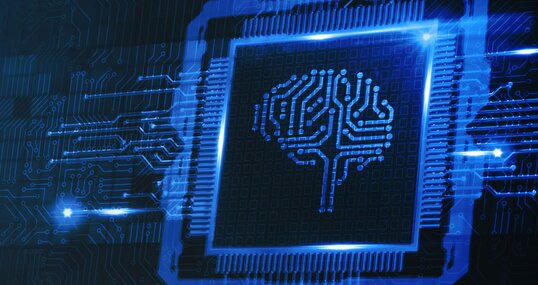
AI & Data Science

Revolutionizing the Logistics Industry
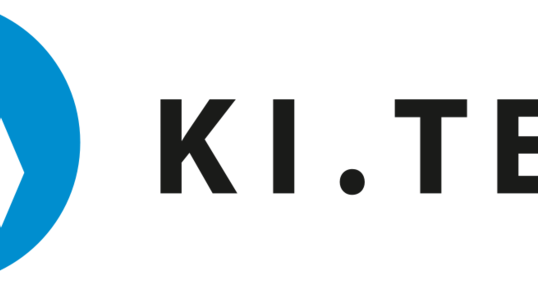
Interactive online portal identifies suitable employees
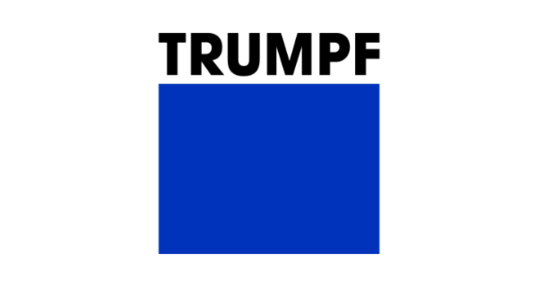
Standardized data management creates basis for reporting
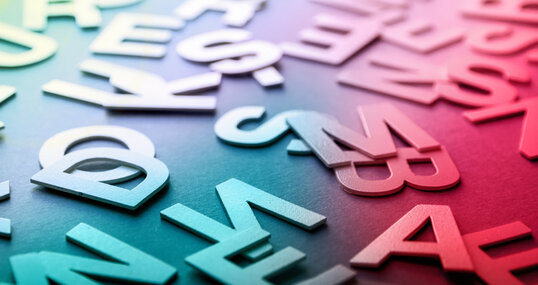
Using NLP libraries for post-processing
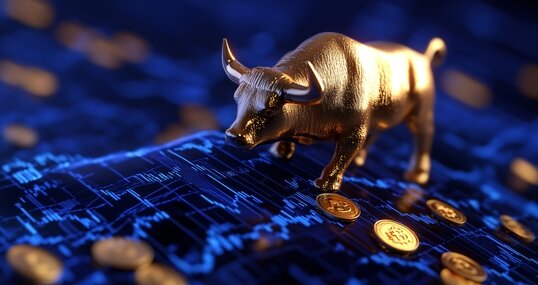
Artificial Intelligence in Treasury Management
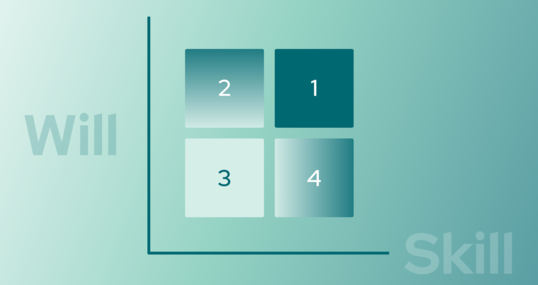
Using a Skill/Will matrix for personal career development
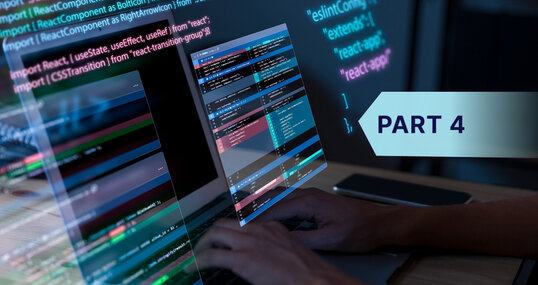
Building A Shell Application for Micro Frontends | Part 4
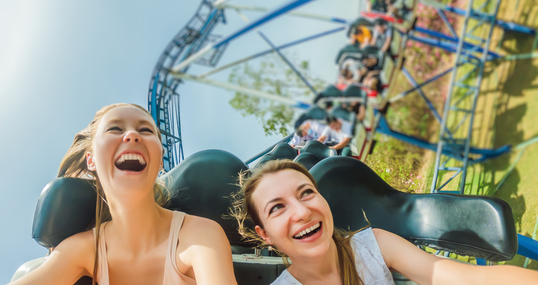
Flexibility in the data evaluation of a theme park
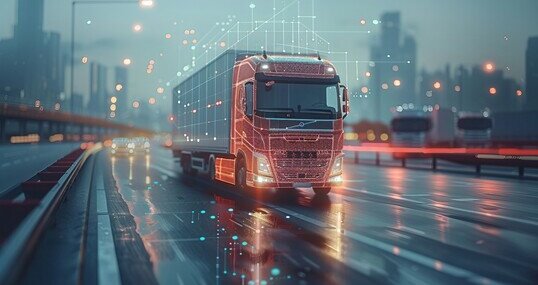
Automated Planning of Transport Routes
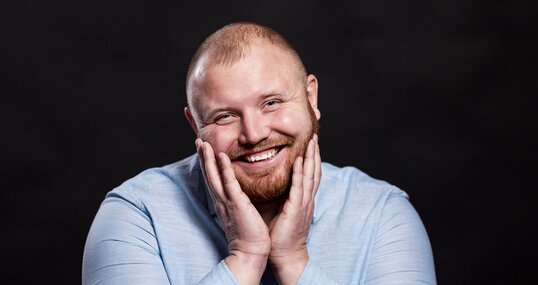
Advanced Admin Trial
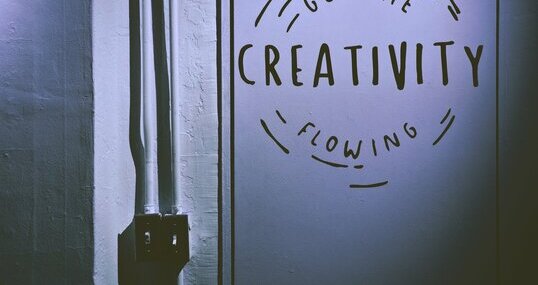
Innovation Incubator at TIMETOACT GROUP Austria
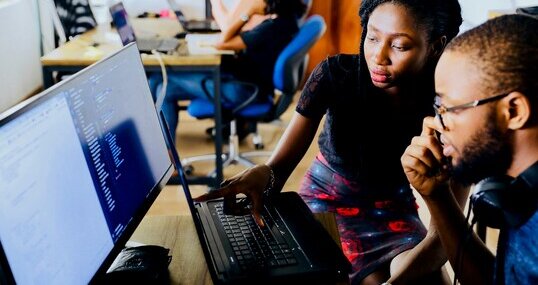
Innovation Incubator Round 1
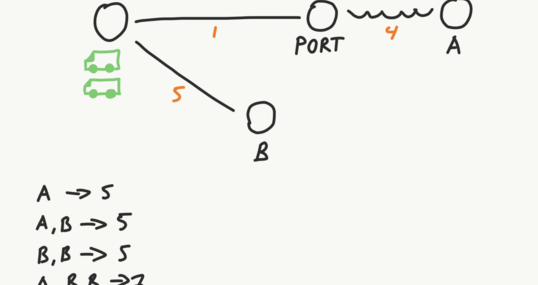
Announcing Domain-Driven Design Exercises
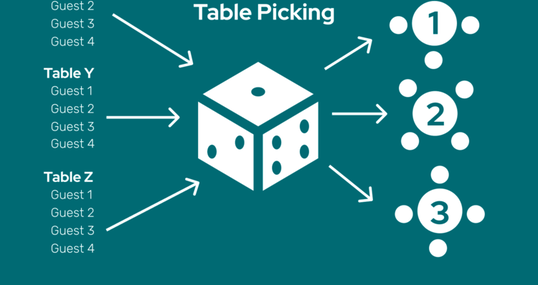
The Power of Event Sourcing
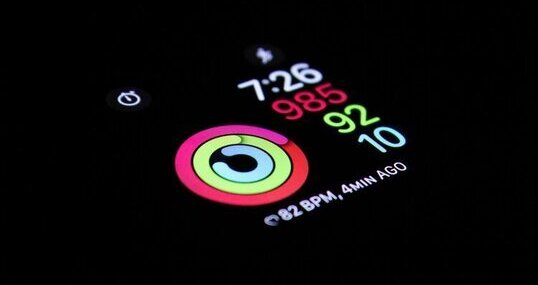
Tracing IO in .NET Core
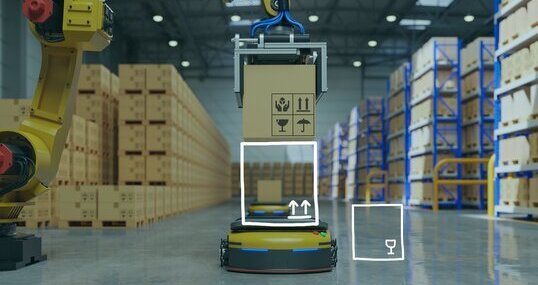
Consistency and Aggregates in Event Sourcing
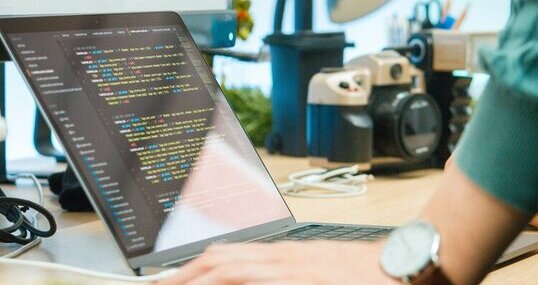
My Workflows During the Quarantine
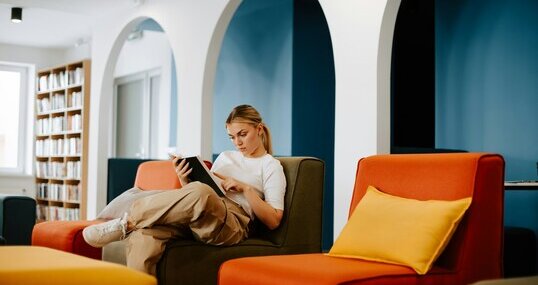
Learning + Sharing at TIMETOACT GROUP Austria
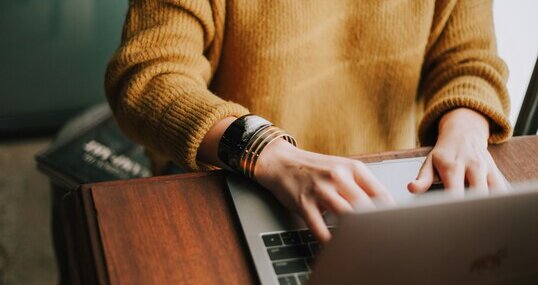
My Weekly Shutdown Routine
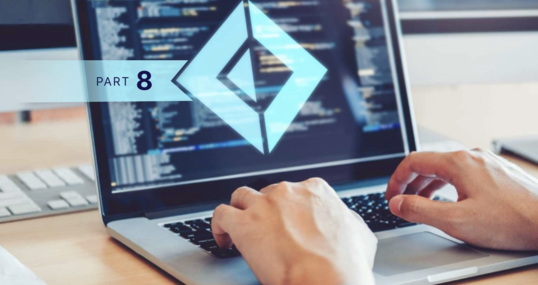
Introduction to Functional Programming in F# – Part 8

Introduction to Functional Programming in F# – Part 9

Introduction to Functional Programming in F# – Part 10
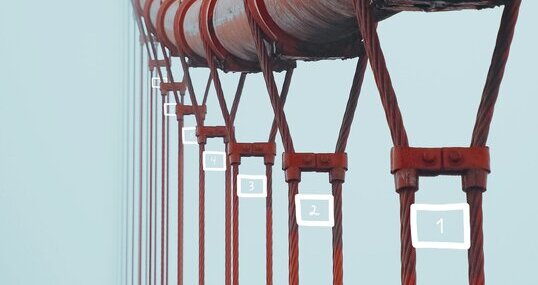
Process Pipelines
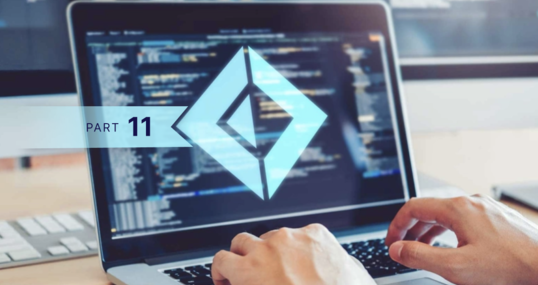
Introduction to Functional Programming in F# – Part 11

Introduction to Functional Programming in F# – Part 12
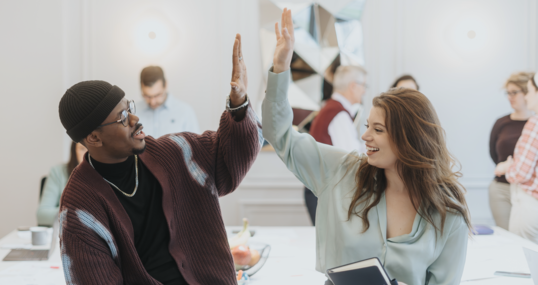
ADRs as a Tool to Build Empowered Teams
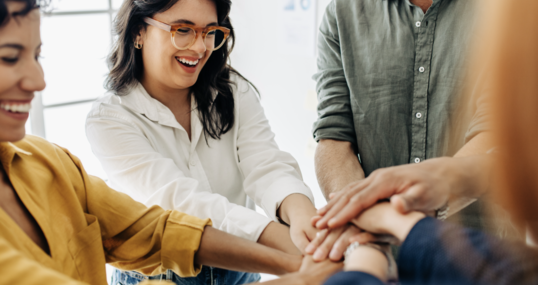
Why Was Our Project Successful: Coincidence or Blueprint?
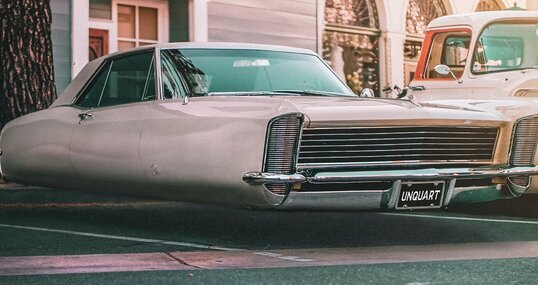
They promised it would be the next big thing!
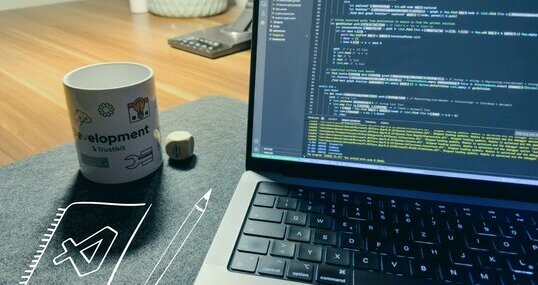
Creating solutions and projects in VS code
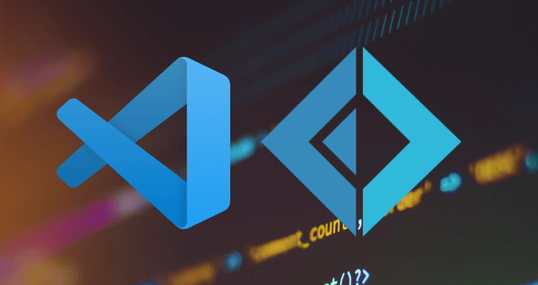
Using Discriminated Union Labelled Fields
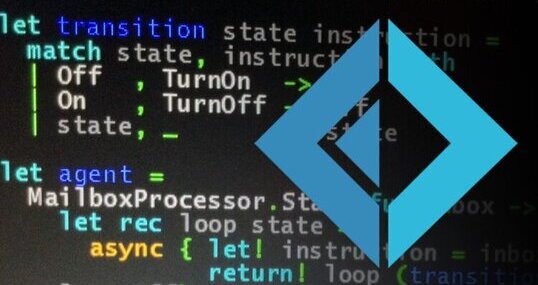
Ways of Creating Single Case Discriminated Unions in F#
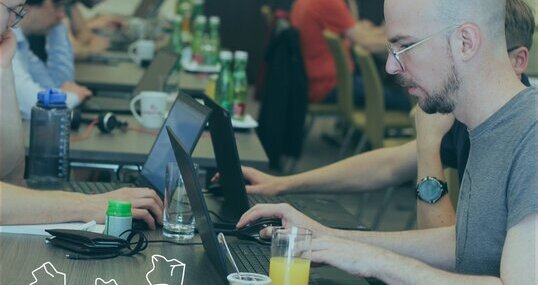
Part 1: TIMETOACT Logistics Hackathon - Behind the Scenes

Understanding F# Type Aliases
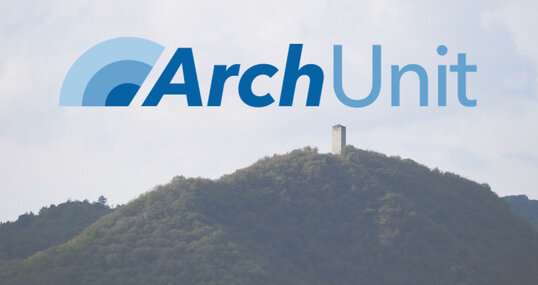
Isolating legacy code with ArchUnit tests
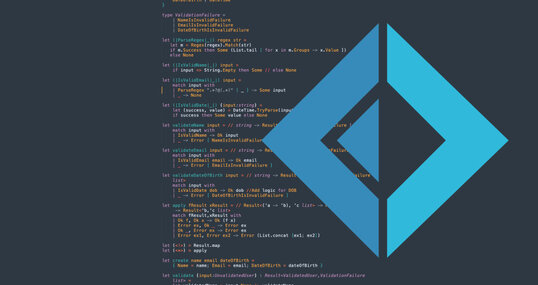
Understanding F# applicatives and custom operators
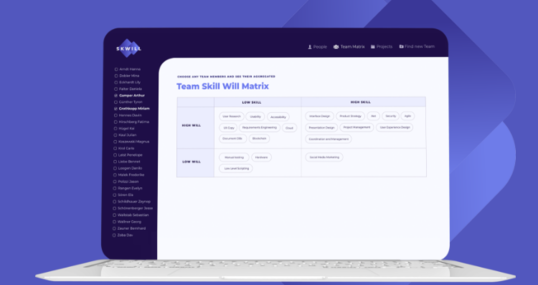
From the idea to the product: The genesis of Skwill
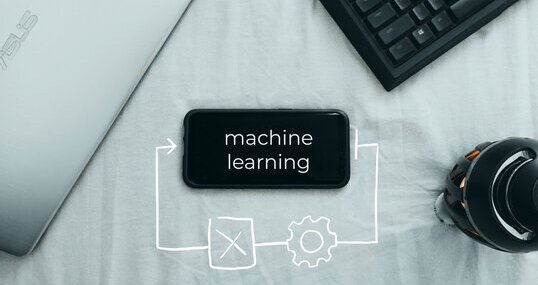
Creating a Cross-Domain Capable ML Pipeline
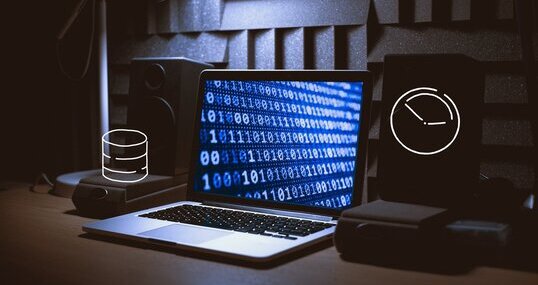
State of Fast Feedback in Data Science Projects
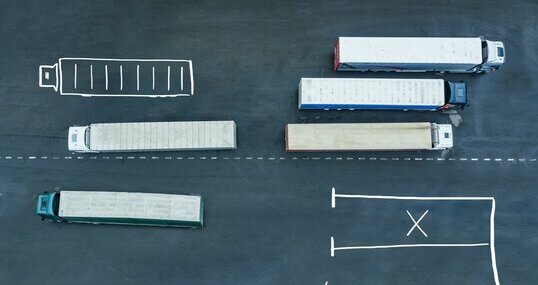
Part 2: Detecting Truck Parking Lots on Satellite Images
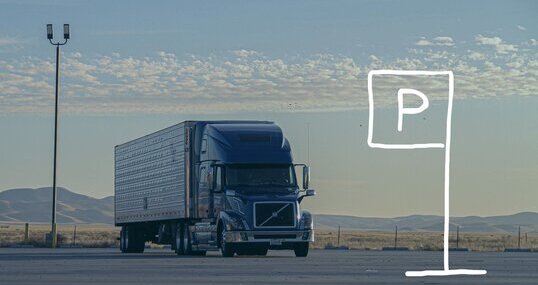
Part 1: Detecting Truck Parking Lots on Satellite Images
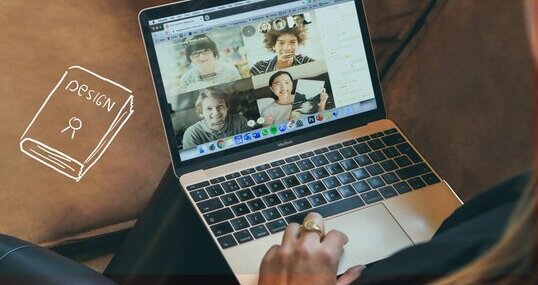
5 lessons from running a (remote) design systems book club

Introduction to Functional Programming in F# – Part 2
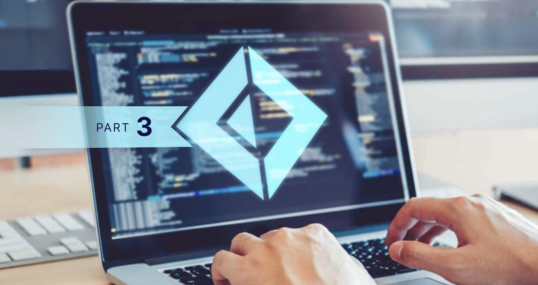
Introduction to Functional Programming in F# – Part 3

Introduction to Functional Programming in F# – Part 4
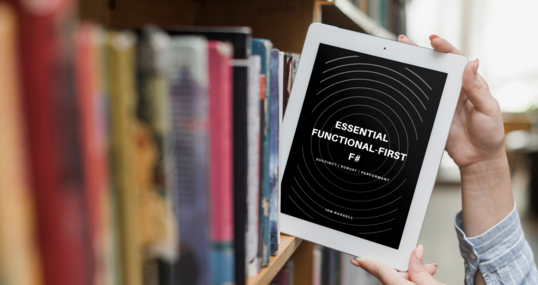
So, I wrote a book

Introduction to Functional Programming in F# – Part 7
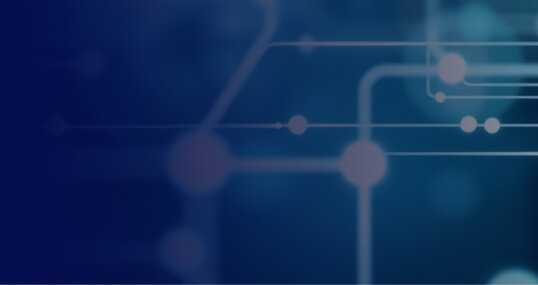
How we discover and organise domains in an existing product
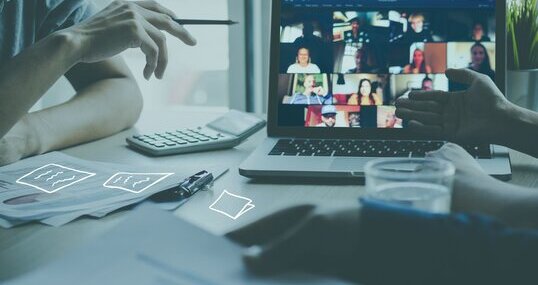
Running Hybrid Workshops
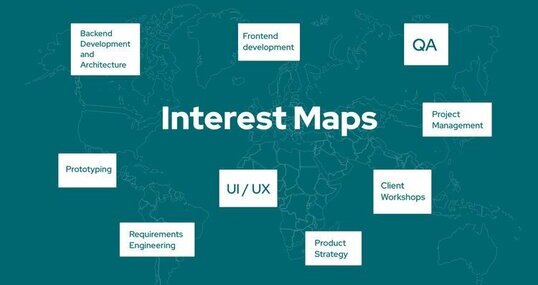
7 Positive effects of visualizing the interests of your team
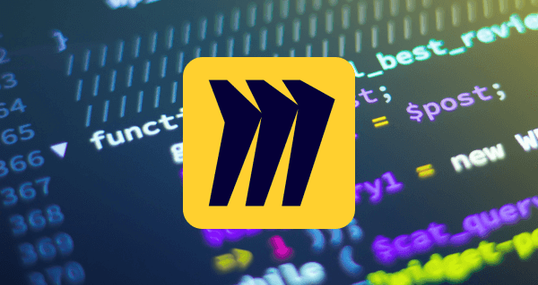
How to gather data from Miro

Designing and Running a Workshop series: An outline

Designing and Running a Workshop series: The board
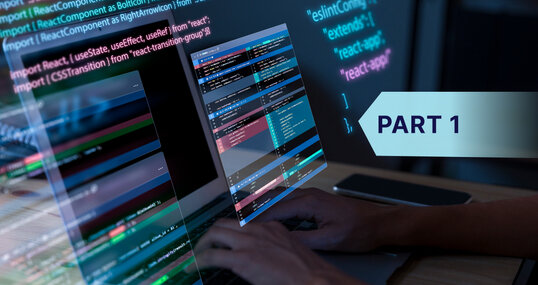
Composite UI with Design System and Micro Frontends
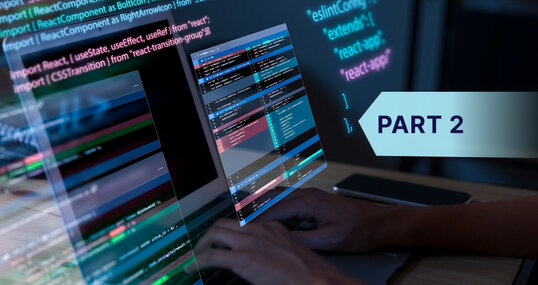
Building and Publishing Design Systems | Part 2
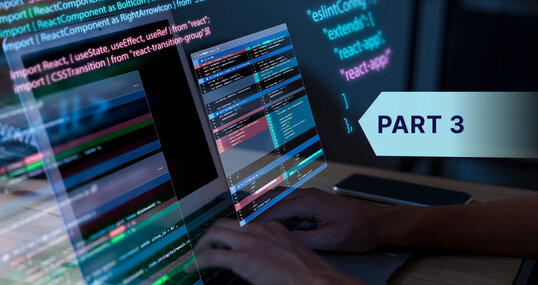
Building a micro frontend consuming a design system | Part 3
